WORKSTREAMS
Data Science & Discovery
Using novel data and methods to enhance our understanding of child and adolescent mental health.
Enriching electronic health records for research
Studying de-identified electronic health records, for example via Clinical Record Interactive Search (CRIS), helps us understand children and young people’s needs, diagnoses, and treatment in new ways. Read more about our use of CRIS on the Clinical & Population Analytics page. Typed clinical notes, i.e., the notes that clinicians take while seeing patients, are a particularly rich and nuanced source of information in electronic health records. However, it is difficult to analyse them, given their length, variability, and complexity.
We use Natural Language Processing (NLP) methods to address this problem. NLP is an automated way of extracting information from large volumes of text. For example, we have developed NLP tools to identify mentions of self-harm in clinical notes, allowing us to study self-harm risk in young people. Another NLP tool has helped us study the relationship between bullying and suicidality among young people with autism. Another problem we are addressing using NLP is the accuracy of recorded diagnoses. In electronic health records, clinicians usually record diagnoses in structured fields. But these fields are not always completed accurately, or at all.
Hence, we have started using Medical Concept Annotation Toolkit (MedCAT), which employs machine learning and artificial intelligence, to extract diagnostic information from typed clinical notes. This will help us understand the treatment pathways of children and adolescents with different mental health diagnoses.
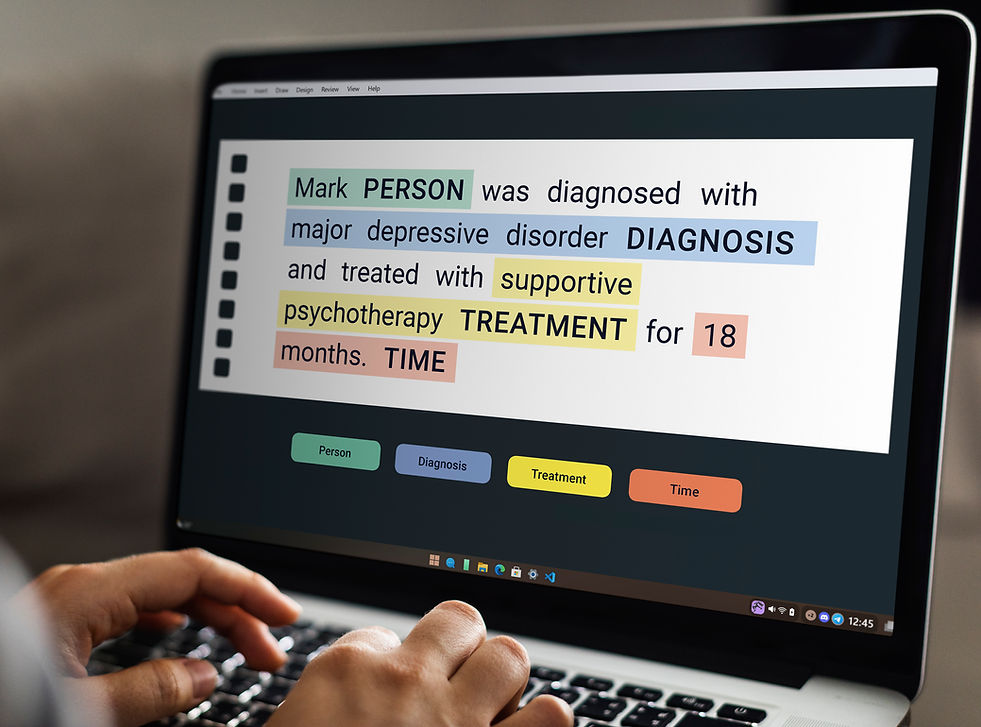
Using novel data
Our lab is exploring how we can use new methods – and new types of data – to gain insights into child and adolescent mental health. For example, we are developing an artificial intelligence algorithm to analyse audio data of mothers talking about their children. This algorithm is designed to detect ‘expressed emotion’, i.e., the emotions a mother feels about her child. We know that parents’ expressed emotion is related to mental health in their children. So, if successful, this algorithm could be used by clinicians as an assessment tool to help identify children and families who need mental health and parenting support.

Research Publications
Digital Lab Workstreams
High impact, design-led translational initiatives aimed to transform child mental health services across the NHS and the rest of the world.